Written by: Marko Maričić
Transportation | 5 mins read
– On-demand transportation services could be the key to alleviating traffic congestion in cities for various activities. Allowing travelers to choose when and where to get picked up can play a key role in convincing private car users to opt for more efficient modes of transport. On-demand services can also enlighten travelers to opt for shared transport services such as bike, car, or ride-sharing; such modes could be vital to reducing the number of vehicles on the road.
People are usually acquainted with bike-sharing but confuse the latter two modes of sharing. Car sharing involves travelers accessing and driving a vehicle that could be picked up anywhere (SIXT share, Greenwheels) while ride-sharing (also known as ride pooling) allows multiple travelers that are going the same direction to share a vehicle that already has a driver, either human or autonomous.
On-Demand Transport Services
Allowing travelers to choose when and where to get picked up can play a key role in convincing private car users to opt for more efficient modes of transport.Focusing on ride pooling, currently services such as UberPool match multiple trips into a single ride where the respective travelers are offered a discount for pooling due to longer travel times and the discomfort of sharing (when compared to a private hailed ride). Ideally, the operator offers a discount that matches the reduction in operating costs due to pooling.
Improving ride-pooling for the traveler and the operator
Now, research shows that incorporating pick-up and drop-off (PUDO) points to a pooled ride where travelers could walk to/from could further improve existing ride pooling services. Undoubtedly, the route optimization problem of ride pooling with PUDO is extremely complex and requires sophisticated algorithms to solve. Fortunately, extensive research already exists on the optimization problem itself but there is a knowledge gap on the performance of ride pooling and its level of service when subject to various pricing configurations and service settings. This was what I investigated for my MSc thesis: “The effects of pricing and service configurations on a ride-pooling service with PUDO points”.
Ride-Pooling with PUDO
The route optimization problem of ride pooling with PUDO is extremely complex and requires sophisticated algorithms to solve.During my MSc thesis, I built upon an existing algorithm, ExMAS, that matches trips into attractive pooled rides by introducing PUDO points to these rides. The pricing of ride pooling services is one of the main parameters in controlling the attractiveness of the services. In theory, PUDO ride pooling should be cheaper than door-to-door ride pooling which should be cheaper than private ride-hailing.
Another way of controlling the performance is through a service setting, which is a weighted parameter on the total cost function that helps select a route given a set of PUDO points. The image below shows the possible routes that can be controlled with some service settings, the resulting route resembles door-to-door ride pooling when Alpha is 0 as we put the weight only on the travelers in a ride where minimal walking time is invoked. On the contrary, setting Alpha closer to 1, we expect a route with longer walking times but minimal detours as we put the weight almost completely on the operator.
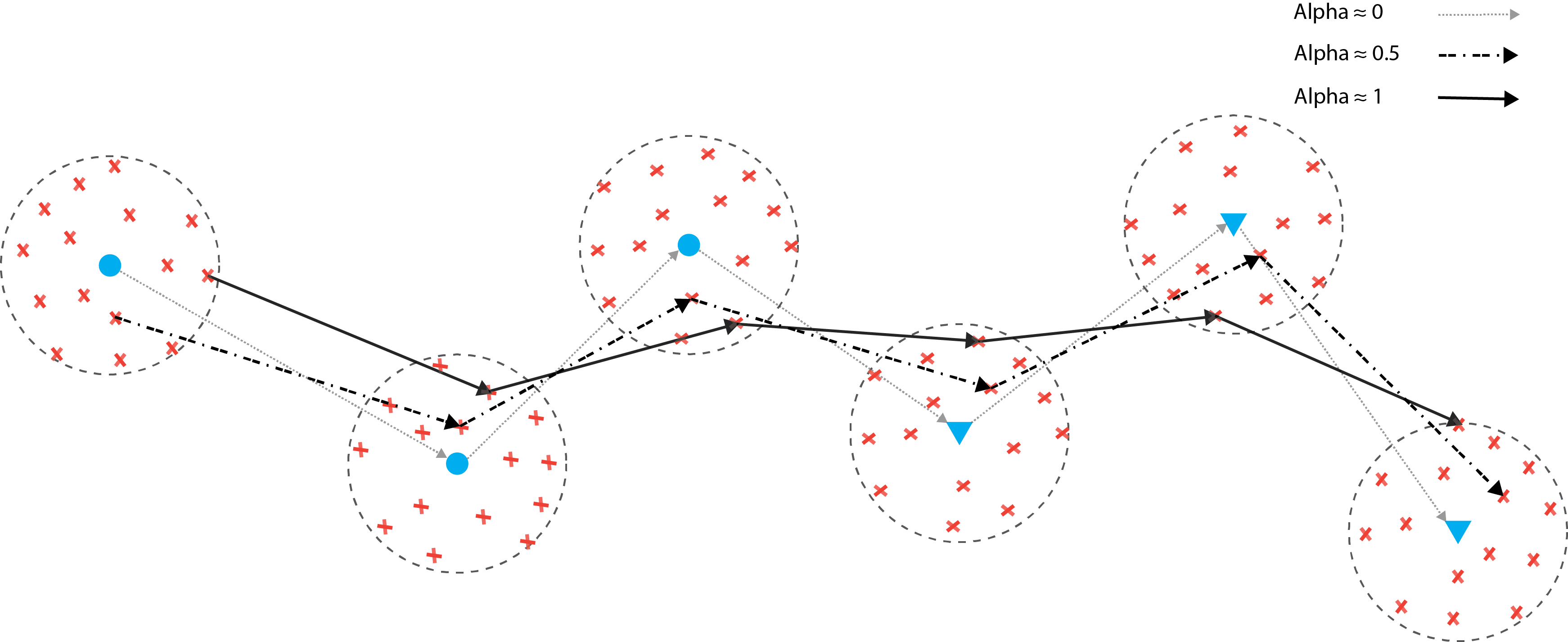
Case Study: Ride-pooling with pick-up and drop-off points in Amsterdam
I conducted my experimentation in the context of Amsterdam under various pricing configurations of PUDO and door-to-door ride pooling along with differing service settings. In this case, the price of the two modes is noted as discounts with respect to the base fare of private ride-hailing (I.e., 30% off the original fare.)
The image below can give you an idea of the resulting optimization of the pooled route with PUDO. The orange and pink lines respectively indicate door-to-door rides with two and four travelers while the new optimized route is due to PUDO in green.
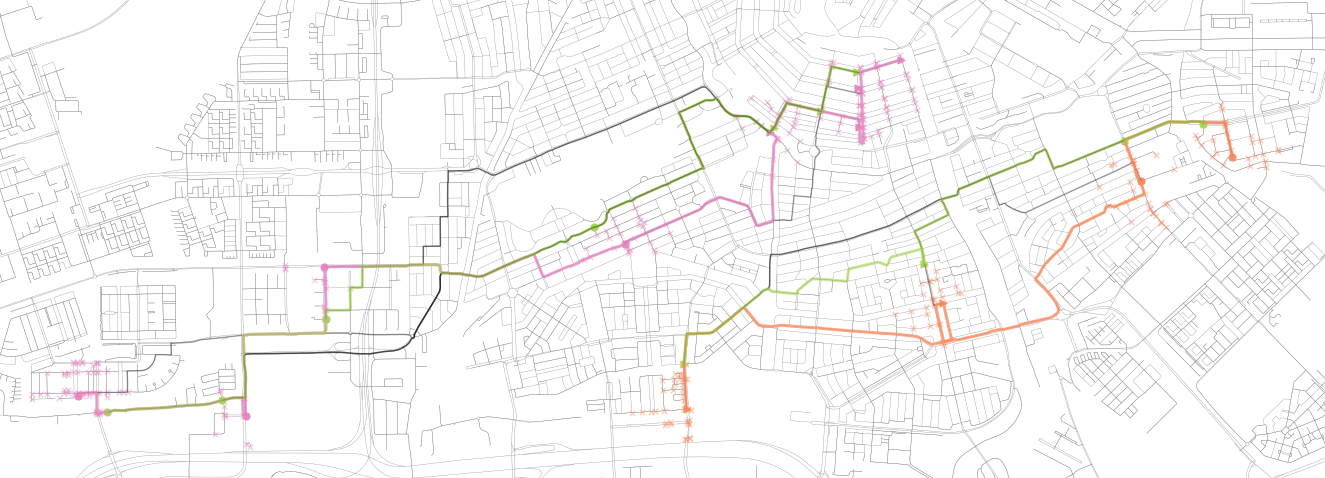
Findings from the study and its implications
Splitting KPIs into different levels allows for a clearer understanding of why a model or software performs the way it does. Now, I could summarize the quantitative results of my research in this blog, but I think you would get a clearer picture by simply reading Sections 3 and 4 of my full thesis or as an extended abstract. We should instead ask, how does the topic of this thesis apply to current and future projects at Ab Ovo?
Utilizing KPIs on a multi-level basis is a very efficient and essential method of characterizing the performance of almost any project. Aggregate KPIs allow you to understand the benefits and drawbacks of certain parameters on a whole system but implementing disaggregate KPIs will allow you to understand why these system-wide indicators occur. A clearer understanding can be formed of how a system operates, which is beneficial for both developers and clients.
Regarding future projects, I believe that the topic of human mobility is quintessential in society and fundamental to the development of society. Such services are constantly being improved where efficient and effective automation of such systems is paramount. However, instead of creating our own transportation, we could partner with companies already busy with such projects and help them improve their services using multi-level KPIs.
More information
For more information, please contact Marko Maričić via marko.maricic@ab-ovo.com or request a discovery session to learn more about ride-pooling and optimizing on-demand transportation services.
Marko Maricic completed his MSc in Transport & Planning in TU Delft in November 2021. His thesis allowed him to gain valuable experience with data handling and analysis using Python; the findings from his research gained interest in academia as he was invited to present his research at a transportation research conference (hEART2022). Marko now works as a Developer in the Supply Chain & Analytics branch of Ab Ovo where he gains valuable experience on projects related to schedule optimization, data management, and image recognition.