Current global developments force organizations to rethink their supply chain. COVID-19 increased trade barriers and higher trade and geopolitical tensions take a heavy toll on growth, including the disruption of supply chains. Supply networks designed for low-cost and minimal inventory pose a major risk and refocus on resilience is required. Furthermore, sustainability and digitization are important for boards of directors to transform to an efficient, digital and sustainable organization.
We believe leveraging the power of data and translating information to actionable insights help organizations to become data-driven, and to optimize and improve supply chain resilience.
While every supply chain is unique, we have researched 50+ supply chain use cases to help supply chains become more data-driven. These are Proven use cases with measurable results, including predictive maintenance, real-time scheduling and self-learning demand planning. By combining emerging cloud technology, extensive business knowledge and larger sets of supply chain data, value-driven analytics is within reach.
To help organizations start or expand the usage of data analytics in advanced decision making, Ab Ovo delivers end-to-end supply chain analytics.
Supply Chain Analytics
We provide solutions and services that facilitates hundreds of planners with data-driven decision makingSupply Chain Analytics
There are multiple ways of delivering analytics. Depending on the situation at hand, different project methodologies and technologies are applied. For large organizations with extensive planning processes and challenges, we develop and deliver scalable advanced planning & scheduling solutions (APS). These solutions facilitate hundreds of planners with daily data-driven decision making.
In the last few years, however, analytics has become more widely available through emerging technologies such as big data, cloud technologies and analytical languages like Python or R. This standardized, partly open source knowledge, paves the way for a truly value-driven/agile way of implementing advanced analytics. First results are achieved in weeks. With full use case implementation, results are achieved around three months. Never has analytics been so tangible, leading to concrete business cases and measurable results that support in decision making. We drive supply chain decision processes with analytics.

We have specifically chosen for analytics in the supply chain because:
- We have over twenty years of experience in implementing decision support tools in this sector and are experienced subject matter experts.
- Our experts and teams, including more than hundred operations research experts, have extensive business knowledge about operational processes in manufacturing/rail/logistics/aviation and maritime.
- Global developments force companies to rethink their supply chains and although companies have marketing and finance analytics already successfully implemented, companies are struggling to get value from models and algorithms to optimize their supply chains.
Data science as an all-purpose word often refers to what we call “predictive analytics”. Predictive analytics is using data to recognize patterns in order to predict the future or what is likely to happen. This in itself can be beneficial, but we strongly believe every prediction in a supply chain lands in a decision process with many bottlenecks and constraints.
For example, in marketing analytics we predict that a certain customer is interested in product A. This prediction is quite easily translated into the decision of showing an advertisement of product A. In a supply chain we can also predict that a certain resource (e.g. a train, truck or an airplane) will need maintenance (this is what we call predictive maintenance). However, in order to schedule this triggered maintenance alert, we must find different available resources (workshop/people) and plan the logistics, service planning and total cost of maintenance.
Hence, a prediction in itself is difficult to implement in a supply chain. We also need analytics to suggest actions based on these predictions. This is what Ab Ovo calls “prescriptive analytics”. And the synergy between predictive and prescriptive analytics is where the true analytical value lies for supply chains. The combination is what is needed to perform business process analytics.
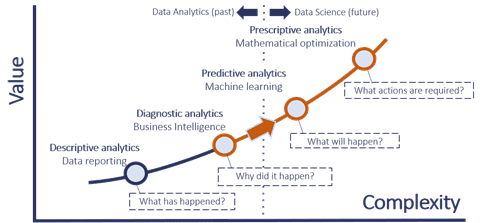
Supply Chain Analytics
We include change management and governance into analytics implementation projects in order to ensure a good fit and acceptance & adaptation on all levelsEnd-To-End
From experience, we know that successfully implementing business process analytics also needs translators and embedding in the existing organization, IT and processes. It is crucial to analyze the whole business process in order to connect predictive and prescriptive analytics to decision makers. Two worlds with their own “language”, so almost literal translation is a necessity.
On top of that, having a good fit with current processes and the technology landscape is required to embed analytics into the organization. Many analytics companies underestimate the embedding process, as the prediction model outcomes are beneficial already on itself. But we include change management and governance into analytics implementation projects in order to ensure a good fit and acceptance & adaptation on all levels.
Supply Chain Analytics
We love working on complex puzzles such as workforce rostering, advanced demand forecasting or building an algorithmUse Cases
Business process analytics can be achieved by a wide range of use cases. We have implemented many use cases combining different analytics and technologies in the last 20 years. We love working on complex puzzles such as workforce rostering, advanced demand forecasting, or building an algorithm for contract evaluation in the childcare industry.
Other examples include an optimization algorithm for Lufthansa Cargo. In it, based on predicted shipments, we optimize the handling of shipments at airports by allocating the best working stations for each of the 70,000 shipments daily. With this automated way of using algorithms, we see an estimated increase in handling efficiency increase of around 12% which leads to an average decrease in throughput time of around 8%. Customer service levels go up while handling and storage costs decrease. Data-driven decisions in the aviation sector prove their value here.
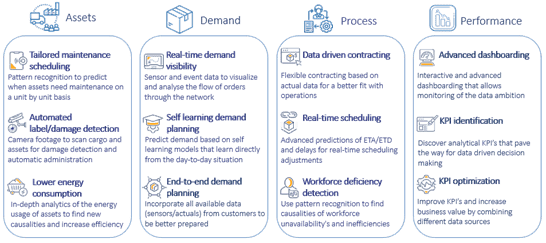
For more information, please contact us or check our use cases.